Sentiment Analysis On Twitter Social Media Using Naїve Bayes Classifier With Swarm Particle Selection Feature Optimization And Term Frequency
Abstract
Full Text:
PDFReferences
Rahman, M.; Islam, M.N. Exploring the performance of ensemble machine learning classifiers for sentiment analysis of covid-19 tweets. In Sentimental Analysis and Deep Learning; Springer: Singapore, 2022; pp. 383–396. [Google Scholar]
Chintalapudi, N.; Battineni, G.; Amenta, F. Sentimental analysis of COVID-19 tweets using deep learning models. Infect. Dis. Rep. 2021, 13, 32. [Google Scholar] [CrossRef] [PubMed]
Mishra, R.K.; Urolagin, S.; Jothi, J.A.; Neogi, A.S.; Nawaz, N. Deep learning-based sentiment analysis and topic modeling on tourism during Covid-19 pandemic. Front. Comput. Sci. 2021, 3, 775368. [Google Scholar] [CrossRef]
Costola, M.; Nofer, M.; Hinz, O.; Pelizzon, L. Machine Learning Sentiment Analysis, COVID-19 News and Stock Market Reactions; (No. 288), SAFE Working Paper; SAFE: Frankfurt am Main, Germany, 2020. [Google Scholar]
Ebadi, A.; Xi, P.; Tremblay, S.; Spencer, B.; Pall, R.; Wong, A. Understanding the temporal evolution of COVID-19 research through machine learning and natural language processing. Scientometrics 2021, 126, 725–739. [Google Scholar] [CrossRef] [PubMed]
Chandrasekaran, R.; Mehta, V.; Valkunde, T.; Moustakas, E. Topics, trends, and sentiments of tweets about the COVID-19 pandemic: Temporal infoveillance study. J. Med. Internet Res. 2020, 22, 1–12. [Google Scholar] [CrossRef] [PubMed]
Khan, R.; Shrivastava, P.; Kapoor, A.; Tiwari, A.; Mittal, A. Social media analysis with AI: Sentiment analysis techniques for the analysis of twitter covid-19 data. Crit. Rev. 2020, 7, 2761–2774. [Google Scholar]
Naseem, U.; Razzak, I.; Khushi, M.; Eklund, P.W.; Kim, J. COVIDSenti: A large-scale benchmark Twitter data set for COVID-19 sentiment analysis. IEEE Trans. Comput. Soc. Syst. 2021, 8, 1003–1015. [Google Scholar] [CrossRef]
Alamoodi, A.H.; Zaidan, B.B.; Zaidan, A.A.; Albahri, O.S.; Mohammed, K.I.; Malik, R.Q.; Almahdi, E.M.; Chyad, M.A.; Tareq, Z.; Alaa, M. Sentiment analysis and its applications in fighting COVID-19 and infectious diseases: A systematic review. Expert Syst. Appl. 2021, 167, 114155. [Google Scholar] [CrossRef]
Nemes, L.; Kiss, A. Social media sentiment analysis based on COVID-19. J. Inf. Telecommun. 2021, 5, 1–15. [Google Scholar] [CrossRef]
Refbacks
- There are currently no refbacks.

This work is licensed under a Creative Commons Attribution 4.0 International License.
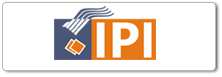
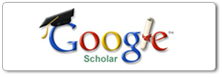