Community Analysis of the Twitter Application on the COVID-19 Pandemic Phenomenon Based on an Artificial Intelligence System
Abstract
The COVID-19 outbreak is a disastrous event that has elevated many psychological problems such as lack of employment and depression given abrupt social changes. Simultaneously, psychologists and social scientists have drawn considerable attention towards understanding how people express their sentiments and emotions during the pandemic. With the rise in COVID-19 cases with strict lockdowns, people expressed their opinions publicly on social networking platforms. This provides a deeper knowledge of human psychology at the time of disastrous events. By applying user-produced content on social networking platforms such as Twitter, the sentiments and views of people are analyzed to assist in introducing awareness campaigns and health intervention policies. The modern evolution of artificial intelligence (AI) and natural language processing (NLP) mechanisms has revealed remarkable performance in sentimental analysis (SA). This study develops a new Marine Predator Optimization with Natural Language Processing for Twitter Sentiment Analysis (MPONLP-TSA) for the COVID-19 Pandemic. The presented MPONLP-TSA model is focused on the recognition of sentiments that exist in the Twitter data during the COVID-19 pandemic. The presented MPONLP-TSA technique undergoes data preprocessing to convert the data into a useful format. Furthermore, the BERT model is used to derive word vectors. To detect and classify sentiments, a bidirectional recurrent neural network (BiRNN) model is utilized. Finally, the MPO algorithm is exploited for optimal hyperparameter tuning process, and it assists in enhancing the overall classification performance. The experimental validation of the MPONLP-TSA approach can be tested by utilizing the COVID-19 tweets dataset from the Kaggle repository. A wide comparable study reported a better outcome of the MPONLP-TSA method over current approaches.
Full Text:
PDFReferences
Rahman, M.; Islam, M.N. Exploring the performance of ensemble machine learning classifiers for sentiment analysis of covid-19 tweets. In Sentimental Analysis and Deep Learning; Springer: Singapore, 2022; pp. 383–396.
Chintalapudi, N.; Battineni, G.; Amenta, F. Sentimental analysis of COVID-19 tweets using deep learning models. Infect. Dis. Rep. 2021, 13, 32.
Mishra, R.K.; Urolagin, S.; Jothi, J.A.; Neogi, A.S.; Nawaz, N. Deep learning-based sentiment analysis and topic modeling on tourism during Covid-19 pandemic. Front. Comput. Sci. 2021, 3, 7753
Costola, M.; Nofer, M.; Hinz, O.; Pelizzon, L. Machine Learning Sentiment Analysis, COVID-19 News and Stock Market Reactions; (No. 288), SAFE Working Paper; SAFE: Frankfurt am Main, Germany, 2020.
Ebadi, A.; Xi, P.; Tremblay, S.; Spencer, B.; Pall, R.; Wong, A. Understanding the temporal evolution of COVID-19 research through machine learning and natural language processing. Scientometrics 2021, 126, 725–739.
Chandrasekaran, R.; Mehta, V.; Valkunde, T.; Moustakas, E. Topics, trends, and sentiments of tweets about the COVID-19 pandemic: Temporal infoveillance study. J. Med. Internet Res. 2020, 22, 1–12.
Khan, R.; Shrivastava, P.; Kapoor, A.; Tiwari, A.; Mittal, A. Social media analysis with AI: Sentiment analysis techniques for the analysis of twitter covid-19 data. Crit. Rev. 2020, 7, 2761–2774.
Naseem, U.; Razzak, I.; Khushi, M.; Eklund, P.W.; Kim, J. COVIDSenti: A large-scale benchmark Twitter data set for COVID-19 sentiment analysis. IEEE Trans. Comput. Soc. Syst. 2021, 8, 1003–1015.
Alamoodi, A.H.; Zaidan, B.B.; Zaidan, A.A.; Albahri, O.S.; Mohammed, K.I.; Malik, R.Q.; Almahdi, E.M.; Chyad, M.A.; Tareq, Z.; Alaa, M. Sentiment analysis and its applications in fighting COVID-19 and infectious diseases: A systematic review. Expert Syst. Appl. 2021, 167, 114155.
Nemes, L.; Kiss, A. Social media sentiment analysis based on COVID-19. J. Inf. Telecommun. 2021, 5, 1–15.
Cervi, L.; García, F.; Marín-Lladó, C. Populism, Twitter, and covid-19: Narrative, fantasies, and desires. Soc. Sci. 2021, 10, 294.
Rufai, S.R.; Bunce, C. World leaders’ usage of Twitter in response to the COVID-19 pandemic: A content analysis. J. Public Health 2020, 42, 510–516.
Emilio, F. # COVID-19 on twitter: Bots, conspiracies, and social media activism. arXiv 2020, arXiv:2004.09531.
Chaves-Montero, A.; Relinque-Medina, F.; Fernández-Borrero, M.Á.; Vázquez-Aguado, O. Twitter, social services and covid-19: Analysis of interactions between political parties and citizens. Sustainability 2021, 13, 2187.
Jena, P.R.; Majhi, R. Are Twitter sentiments during COVID-19 pandemic a critical determinant to predict stock market movements? A machine learning approach. Sci. Afr. 2023, 19, e01480.
Mostafa, L. Egyptian student sentiment analysis using Word2vec during the coronavirus (Covid-19) pandemic. In Proceedings of the International Conference on Advanced Intelligent Systems and Informatics, Cairo, Egypt, 19–21 October 2020; Springer: Cham, Switzerland, 2020; pp. 195–203.
Chandra, R.; Krishna, A. COVID-19 sentiment analysis via deep learning during the rise of novel cases. PLoS ONE 2021, 16, e0255615.
Mohan, S.; Solanki, A.K.; Taluja, H.K.; Singh, A. Predicting the impact of the third wave of COVID-19 in India using hybrid statistical machine learning models: A time series forecasting and sentiment analysis approach. Comput. Biol. Med. 2022, 144, 105354.
Alkhaldi, N.A.; Asiri, Y.; Mashraqi, A.M.; Halawani, H.T.; Abdel-Khalek, S.; Mansour, R.F. Leveraging Tweets for Artificial Intelligence Driven Sentiment Analysis on the COVID-19 Pandemic. Healthcare 2022, 10, 910.
Alorini, G.; Rawat, D.B.; Alorini, D. LSTM-RNN Based Sentiment Analysis to Monitor COVID-19 Opinions using Social Media Data. In Proceedings of the ICC 2021-IEEE International Conference on Communications, Montreal, QC, Canada, 14–23 June 2021; IEEE: Piscataway, NJ, USA, 2021; pp. 1–6.
Hossain, G.S.; Assaduzzaman, S.; Mynoddin, M.; Sarker, I.H. A Deep Learning Approach for Public Sentiment Analysis in COVID-19 Pandemic. In Proceedings of the 2022 2nd International Conference on Intelligent Technologies (CONIT), Hubli, India, 24–26 June 2022; pp. 1–6.
Yang, L.; Li, Y.; Wang, J.; Sherratt, R.S. Sentiment analysis for E-commerce product reviews in Chinese based on sentiment lexicon and deep learning. IEEE Access 2020, 8, 23522–2354.
Zhou, G.B.; Wu, J.; Zhang, C.L.; Zhou, Z.H. Minimal gated unit for recurrent neural networks. Int. J. Autom. Comput. 2016, 13, 226–234.
Faramarzi, A.; Heidarinejad, M.; Mirjalili, S.; Gandomi, A.H. Marine Predators Algorithm: A Nature-inspired Metaheuristic. Expert Syst. Appl. 2020, 152, 113377
Al-Qaness, M.A.; Ewees, A.A.; Fan, H.; Abualigah, L.; Abd Elaziz, M. Marine predators algorithm for forecasting confirmed cases of COVID-19 in Italy, USA, Iran and Korea. Int. J. Environ. Res. Public Health 2020, 17, 3520.
Sentiment Analysis of COVID-19 Related Tweets. Available online: https://www.kaggle.com/competitions/sentiment-analysisof-covid-19-related-tweets/data?select=validation.csv (accessed on 11 December 2022).
Singh, C.; Imam, T.; Wibowo, S.; Grandhi, S. A deep learning approach for sentiment analysis of COVID-19 reviews. Appl. Sci. 2022, 12, 3709.
Refbacks
- There are currently no refbacks.

This work is licensed under a Creative Commons Attribution 4.0 International License.
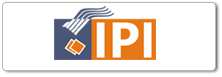
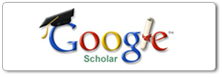