Measuring Public Interest in Online Social Media
Abstract
Full Text:
PDFReferences
Adamic L, Glance N. 2005. The political blogosphere and the 2004 US election: divided they blog. In: LinkKDD’05: proceedings of the 3rd international workshop on Link discovery. 36-43
An J, Quercia D, Cha M, Gummadi K, Crowcroft J. 2014. Sharing political news: the balancing act of intimacy and socialization in selective exposure. EPJ Data Science 3 Article 12
Bakshy E, Messing S, Adamic L. 2015. Exposure to ideologically diverse news and opinion on facebook. Science
Bakshy E, Rosenn I, Marlow C, Adamic L. 2012. The role of social networks in information diffusion. In: Proceedings of the 21st international conference on world wide web, WWW’12. New York: ACM. 519-528
Baron J. 2000. Thinking and deciding (3rd edition). New York: Cambridge University Press.
Conover M, Ratkiewicz J, Francisco M, Gonçalves B, Flammini A, Menczer F. 2011. Political polarization on twitter. In: Proceedings of the 5th international AAAI conference on weblogs and social media (ICWSM).
Conover MD, Gonçalves B, Flammini A, Menczer F. 2012. Partisan asymmetries in online political activity. EPJ Data Science 1 Article 6
Doris-Down A, Versee H, Gilbert E. 2013. Political blend: an application designed to bring people together based on political differences. In: Proceedings of the 6th international conference on communities and technologies. New York. ACM. 120-130
Flaxman S, Goel S, Rao JM. 2013. Ideological segregation and the effects of social media on news consumption. In: SSRN Scholarly Paper ID 2363701. New York: Social Science Research Network.
Fortunato S, Flammini A, Menczer F, Vespignani A. 2006. Topical interests and the mitigation of search engine bias. Proceedings of the National Academy of Sciences of the United States of America 103(34):12684-12689
Gilbert E, Bergstrom T, Karahalios K. 2009. Blogs are echo chambers: blogs are echo chambers. In: Proceedings of HICSS. Piscataway. IEEE. 1-10
Google. 2009a. Introducing google social search: I finally found my friend’s New York blog! Available at http://googleblog.blogspot.com/2009/10/introducing-google-social-search-i.html (accessed 6 January 2015)
Google. 2009b. Personalized search for everyone. Available at http://googleblog.blogspot.com/2009/12/personalized-search-for-everyone.html (accessed 25 August 2014)
Graells-Garrido E, Lalmas M, Quercia D. 2013. Data portraits: connecting people of opposing views. Technical Report. preprint
Grevet C, Terveen LG, Gilbert E. 2014. Managing political differences in social media. In: Proceedings of the 17th ACM conference on computer supported cooperative work & social computing. New York. ACM. 1400-1408
Hart W, Albarracín D, Eagly AH, Brechan I, Lindberg MJ, Merrill L. 2009. Feeling validated versus being correct: a meta-analysis of selective exposure to information. Psychological Bulletin 135(4):555-588
Hosanagar K, Fleder D, Lee D, Buja A. 2013. Will the global village fracture into tribes? Recommender systems and their effects on consumer fragmentation. Management Science 60(4):805-823
Kastenmüller A, Greitemeyer T, Jonas E, Fischer P, Frey D. 2010. Selective exposure: the impact of collectivism and individualism. British Journal of Social Psychology 49(4):745-763
Koutra D, Bennett P, Horvitz E. 2014. Events and controversies: influences of a shocking news event on information seeking. Technical Report. preprint
Matsa KE, Mitchell A. 2014. 8 key takeaways about social media and news. Available at http://www.journalism.org/2014/03/26/8-key-takeaways-about-social-media-and-news/ (accessed 3 September 2014)
McKenzie CR. 2004. Hypothesis testing and evaluation. In: Koehler DJ, Harvey N, eds. Handbook of judgment and decision making. Oxford: Blackwell. 200-219 Chapter 10
Meiss MR, Menczer F, Fortunato S, Flammini A, Vespignani A. 2008. Ranking web sites with real user traffic. In: Proceedings of the 2008 international conference on web search and data mining. New York: ACM. 65-76
Mervis J. 2014. An internet research project draws conservative ire. Science 346(6210):686-687
Mobasher B, Cooley R, Srivastava J. 2000. Automatic personalization based on web usage mining. Communications of the ACM 43(8):142-151
Munson SA, Resnick P. 2010. Presenting diverse political opinions: How and how much. In: Proceedings of the SIGCHI conference on human factors in computing systems. New York: ACM. 1457-1466
Nickerson RS. 1998. Confirmation bias: a ubiquitous phenomenon in many guises. Review of General Psychology 2(2):175-220
Nyhan B, Reifler J. 2010. When corrections fail: the persistence of political misperceptions. Political Behavior 32(2):303-330
Pariser E. 2011. The filter bubble: how the new personalized Web is changing what we read and how we think. London: Penguin.
Refbacks
- There are currently no refbacks.

This work is licensed under a Creative Commons Attribution 4.0 International License.
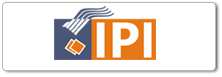
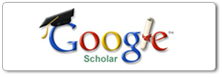