Advanced Persistent Threats (APTs) What You Need to Know with Machine Learning impact
Abstract
Full Text:
PDFReferences
Steffens, T. (2020). Advanced persistent threats. In Attribution of Advanced Persistent Threats: How to Identify the Actors Behind Cyber-Espionage (pp. 3-21). Berlin, Heidelberg: Springer Berlin Heidelberg.
Altun, A., & Yildirim, M. (2022). A research on the new generation artificial intelligence: GPT-3 model. IEEE Access, 10, 12345–12356. https://doi.org/10.1109/ACCESS.2022.9998298.
Zou, Q., Sun, X., Liu, P., & Singhal, A. (2020). An approach for detection of advanced persistent threat attacks. Computer, 53(12), 92-96.
Munagandla, V. B., Vadde, B. C., & Dandyala, S. S. V. (2020). Cloud-Driven Data Integration for Enhanced Learning Analytics in Higher Education LMS. Revista de Inteligencia Artificial en Medicina, 11(1), 279-299.
Xie, Y. X., Ji, L. X., Li, L. S., Guo, Z., & Baker, T. (2021). An adaptive defense mechanism to prevent advanced persistent threats. Connection Science, 33(2), 359-379.
Nersu, S. R. K., Kathram, S. R., & Mandaloju, N. (2020). Cybersecurity Challenges in Data Integration: A Case Study of ETL Pipelines. Revista de Inteligencia Artificial en Medicina, 11(1), 422-439.
Nichols, R. A. (2020). Analysis of Factors to Reduce Advanced Persistent Threat (APT) Exploitation Risk: A Delphi Study. Capella University.
Kathram, S. R., & Nersu, S. R. K. (2020). Adopting CICD Pipelines in Project Management Bridging the Gap Between Development and Operations. Revista de Inteligencia Artificial en Medicina, 11(1), 440- 461.
Khalid, A., Zainal, A., Maarof, M. A., & Ghaleb, F. A. (2021, January). Advanced persistent threat detection: A survey. In 2021 3rd International Cyber Resilience Conference (CRC) (pp. 1-6). IEEE.
Vadde, B. C., Munagandla, V. B., & Dandyala, S. S. V. (2021). Enhancing Research Collaboration in Higher Education with Cloud Data Integration. International Journal of Machine Learning Research in Cybersecurity and Artificial Intelligence, 12(1), 366385.
Kathram, S. R., & Nersu, S. R. K. (2022). Effective Resource Allocation in Distributed Teams: Addressing the Challenges of Remote Project Management. Revista de Inteligencia Artificial en Medicina, 13(1), 615-634.
Brandao, P. R. (2021). Advanced persistent threats (apt)-attribution-mictic framework extension. Journal of Computer Science, 17(5), 470-479.
Nersu, S. R. K., & Kathram, S. R. (2022). Harnessing Federated Learning for Secure Distributed ETL Pipelines. Revista de Inteligencia Artificial en Medicina, 13(1), 592-615.
Steffens, T. (2020). Attribution of Advanced Persistent Threats (pp. 153-164). Springer Berlin Heidelberg.
Mandaloju, N., kumar Karne, V., Srinivas, N., & Nadimpalli, S. V. (2021). Overcoming Challenges in Salesforce Lightning Testing with AI Solutions. ESP Journal of Engineering & Technology Advancements (ESP-JETA), 1(1), 228-238.
Kothamali, P. R., & Banik, S. (2019). Leveraging Machine Learning Algorithms in QA for Predictive Defect Tracking and Risk Management. International Journal of Advanced Engineering Technologies and Innovations, 1(4), 103-120.
de Abreu, S. F., Kendzierskyj, S., & Jahankhani, H. (2020). Attack Vectors and Advanced Persistent Threats. Cyber Defence in the Age of AI, Smart Societies and Augmented Humanity, 267-288.
Banik, S., & Kothamali, P. R. (2019). Developing an End-to-End QA Strategy for Secure Software: Insights from SQA Management. International Journal of Machine Learning Research in Cybersecurity and Artificial Intelligence, 10(1), 125-155.
Khan, M. B. (2020). Advanced persistent threat: Detection and defence. arXiv preprint arXiv:2004.10690.
Kothamali, P. R., & Banik, S. (2019). Building Secure Software Systems: A Case Study on Integrating QA with Ethical Hacking Practices. Revista de Inteligencia Artificial en Medicina, 10(1), 163-191.
Xiang, Z., Guo, D., & Li, Q. (2020). Detecting mobile advanced persistent threats based on large-scale DNS logs. Computers & Security, 96, 101933.
Kothamali, P. R., & Banik, S. (2019). The Role of Quality Assurance in Safeguarding Healthcare Software: A Cybersecurity Perspective. Revista de Inteligencia Artificial en Medicina, 10(1), 192-228.
Kothamali, P. R., & Banik, S. (2020). The Future of Threat Detection with ML. International Journal of Advanced Engineering Technologies and Innovations, 1(2), 133-152.
Myneni, S., Chowdhary, A., Sabur, A., Sengupta, S., Agrawal, G., Huang, D., & Kang, M. (2020). DAPT 2020-constructing a benchmark dataset for advanced persistent threats. In Deployable Machine Learning for Security Defense: First International Workshop, MLHat 2020, San Diego, CA, USA, August 24, 2020, Proceedings 1 (pp. 138-163). Springer International Publishing.
Banik, S., Dandyala, S. S. M., & Nadimpalli, S. V. (2020). Introduction to Machine Learning in Cybersecurity. International Journal of Machine Learning Research in Cybersecurity and Artificial Intelligence, 11(1), 180-204.
Kothamali, P. R., Banik, S., & Nadimpalli, S. V. (2020). Introduction to Threat Detection in Cybersecurity. International Journal of Advanced Engineering Technologies and Innovations, 1(2), 113- 132.
Muqorobin, M., Kusrini, K., & Luthfi, E. T. (2019). Optimasi Metode Naive Bayes Dengan Feature Selection Information Gain Untuk Prediksi Keterlambatan Pembayaran Spp Sekolah. Jurnal Ilmiah SINUS, 17(1), 1-14.
Han, X., Pasquier, T., Bates, A., Mickens, J., & Seltzer, M. (2020). Unicorn: Runtime provenance-based detector for advanced persistent threats. arXiv preprint arXiv:2001.01525.
Kothamali, P. R., Banik, S., & Nadimpalli, S. V. (2021). Feature Engineering for Effective Threat Detection. International Journal of Machine Learning Research in Cybersecurity and Artificial Intelligence, 12(1), 341-358.
Zimba, A., Chen, H., Wang, Z., & Chishimba, M. (2020). Modeling and detection of the multi-stages of Advanced Persistent Threats attacks based on semi-supervised learning and complex networks characteristics. Future Generation Computer Systems, 106, 501-517.
Banik, S., & Dandyala, S. S. M. (2021). Unsupervised Learning Techniques in Cybersecurity. Revista de Inteligencia Artificial en Medicina, 12(1), 384-406.
Kothamali, P. R., & Banik, S. (2021). Data Sources for Machine Learning Models in Cybersecurity. Revista de Inteligencia Artificial en Medicina, 12(1), 358-383.
Kothamali, P. R., Banik, S., & Nadimpalli, S. V. (2020). Challenges in Applying ML to Cybersecurity. Revista de Inteligencia Artificial en Medicina, 11(1), 214-256.
Muqorobin, M. (2021). Analysis Of Fee Accounting Information Systems Lecture At Itb Aas Indonesia In The Pandemic Time Of Covid-19. International Journal of Economics, Business and Accounting Research (IJEBAR), 5(3), 1994-2007.
Tian, W., Du, M., Ji, X., Liu, G., Dai, Y., & Han, Z. (2021). Honeypot detection strategy against advanced persistent threats in industrial internet of things: A prospect theoretic game. IEEE Internet of Things Journal, 8(24), 17372-17381.
Kothamali, P. R., & Banik, S. (2022). Limitations of Signature-Based Threat Detection. Revista de Inteligencia Artificial en Medicina, 13(1), 381-391.
Muqorobin, M., & Rais, N. A. R. (2020, November). Analisis Peran Teknologi Sistem Informasi Dalam Pembelajaran Kuliah Dimasa Pandemi Virus Corona. In Prosiding Seminar Nasional & Call for Paper STIE AAS (Vol. 3, No. 1, pp. 157-168).
Huang, L., & Zhu, Q. (2020). A dynamic games approach to proactive defense strategies against advanced persistent threats in cyber-physical systems. Computers & Security, 89, 101660.
Kothamali, P. R., & Banik, S. (2020). The Future of Threat Detection with ML. International Journal of Advanced Engineering Technologies and Innovations, 1(2), 133-152.
Baksi, R. P., & Upadhyaya, S. J. (2021). Decepticon: a theoretical framework to counter advanced persistent threats. Information Systems Frontiers, 23, 897-913.
Kothamali, P. R., Banik, S., & Nadimpalli, S. V. (2021). Feature Engineering for Effective Threat Detection. International Journal of Machine Learning Research in Cybersecurity and Artificial Intelligence, 12(1), 341-358.
Muqorobin, M., & Rais, N. A. R. (2020). Analysis of the role of information systems technology in lecture learning during the corona virus pandemic. International Journal of Computer and Information System (IJCIS), 1(2), 47-51.
Kothamali, P. R., & Banik, S. (2021). Data Sources for Machine Learning Models in Cybersecurity. Revista de Inteligencia Artificial en Medicina, 12(1), 358-383.
Muqorobin, M., Kusrini, K., Rokhmah, S., & Muslihah, I. (2020). Estimation System For Late Payment Of School Tuition Fees. International Journal of Computer and Information System (IJCIS), 1(1), 1-6.
Li, H., Wu, J., Xu, H., Li, G., & Guizani, M. (2021). Explainable intelligence-driven defense mechanism against advanced persistent threats: A joint edge game and AI approach. IEEE Transactions on Dependable and Secure Computing, 19(2), 757-775.
Muqorobin, M., Rokhmah, S., Muslihah, I., & Rais, N. A. R. (2020). Classification of Community Complaints Against Public Services on Twitter. International Journal of Computer and Information System (IJCIS), 1(1), 7-10.
Muqorobin, M., Hisyam, Z., Mashuri, M., Hanafi, H., & Setiyantara, Y. (2019). Implementasi Network Intrusion Detection System (NIDS) Dalam Sistem Keamanan Open Cloud Computing. Majalah Ilmiah Bahari Jogja, 17(2), 1-9.
Kothamali, P. R., & Banik, S. (2022). Limitations of Signature-Based Threat Detection. Revista de Inteligencia Artificial en Medicina, 13(1), 381-391.
Shang, L., Guo, D., Ji, Y., & Li, Q. (2021). Discovering unknown advanced persistent threat using shared features mined by neural networks. Computer Networks, 189, 107937.
Yang, J., Zhang, Q., Jiang, X., Chen, S., & Yang, F. (2021). Poirot: Causal correlation aided semantic analysis for advanced persistent threat detection. IEEE Transactions on Dependable and Secure Computing, 19(5), 3546-3563.
Muqorobin, M., Apriliyani, A., & Kusrini, K. (2019). Sistem Pendukung Keputusan Penerimaan Beasiswa dengan Metode SAW. Respati, 14(1).
Kothamali, P. R., Mandaloju, N., & Dandyala, S. S. M. (2022). Optimizing Resource Management in Smart Cities with AI. Unique Endeavor in Business & Social Sciences, 1(1), 174-191. https://unbss.com/index.php/unbss/article/view/54
Adeyoola, H. (2021). Advanced Persistent Threat: Detection and Defence (Doctoral dissertation, Bachelor in Science University of Bradford).
Moothedath, S., Sahabandu, D., Allen, J., Clark, A., Bushnell, L., Lee, W., & Poovendran, R. (2020). A game-theoretic approach for dynamic information flow tracking to detect multistage advanced persistent threats. IEEE Transactions on Automatic Control, 65(12), 5248-5263..
Refbacks
- There are currently no refbacks.

This work is licensed under a Creative Commons Attribution 4.0 International License.
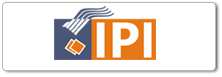
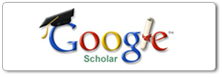